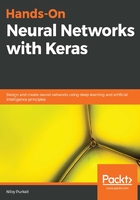
Weight regularization experiments
Simply put, regularizers let us apply penalties to layer parameters during optimization. These penalties are incorporated in to the loss function that the network optimizes. In Keras, we regularize the weights of a layer by passing a kernel_regularizer instance to a layer:
import keras.regularizers
model=Sequential()
model.add(Flatten(input_shape=(28,28)))
model.add(Dense(1024, kernel_regularizer=regularizers.12(0.0001),
activation='relu'))
model.add(Dense(10, activation='softmax'))
As we mentioned previously, we add L2 regularization to both our layers, each with an alpha value of (0.0001). The alpha value of a regularizer simply refers to the transformation that's being applied to each coefficient in the weight matrix of the layer, before it is added to the total loss of our network. In essence, the alpha value is used to multiply each coefficient in our weight matrix with it (in our case, 0.0001). The different regularizers in Keras can be found in keras.regularizers. The following diagram shows how regularization impacts validation loss per epoch on two models that are the same size. One observes that our regularized model is much less prone to overfitting, since the validation loss does not significantly increase as a function of time. On the model without regularization, we can clearly see that this is not the case, and after about seven epochs, the model starts overfitting, and so performs worse on the validation set:
