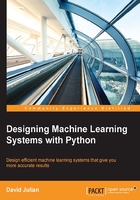
Chapter 2. Tools and Techniques
Python comes equipped with a large library of packages for machine learning tasks.
The packages we will look at in this chapter are as follows:
- The IPython console
- NumPy, which is an extension that adds support for multi-dimensional arrays, matrices, and high-level mathematical functions
- SciPy, which is a library of scientific formulae, constants, and mathematical functions
- Matplotlib, which is for creating plots
- Scikit-learn, which is a library for machine learning tasks such as classification, regression, and clustering
There is only enough space to give you a flavor of these huge libraries, and an important skill is being able to find and understand the reference material for the various packages. It is impossible to present all the different functionality in a tutorial style documentation, and it is important to be able to find your way around the sometimes dense API references. A thing to remember is that the majority of these packages are put together by the open source community. They are not monolithic structures like you would expect from a commercial product, and therefore, understanding the various package taxonomies can be confusing. However, the persity of approaches of open source software, and the fact that ideas are being contributed continually, give it an important advantage.
However, the evolving quality of open source software has its down side, especially for ML applications. For example, there was considerable reluctance on behalf of the Python machine learning user community to move from Python 2 to 3. Because Python 3 broke backwards compatibility; importantly, in terms of its numerical handling, it was not a trivial process to update the relevant packages. At the time of writing, all of the important (well important for me!) packages, and all those used in this book, were working with Python 2.7 or 3x. The major distributions of Python have Python 3 versions with a slightly different package set.